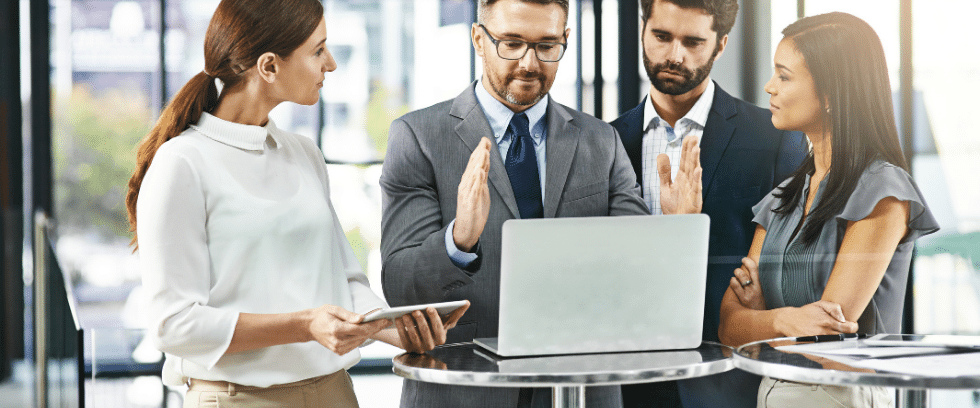
Introduction:
-
- In this document, we are going see the flow of query processing and result caching feature in Amazon Redshift.
The Query Planning And Execution Workflow:
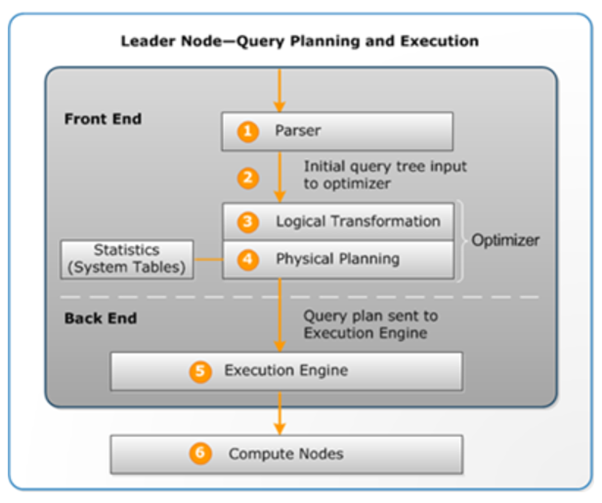
-
- The leader node receives the query and parses the SQL.
- The parser produces an initial query tree that is a logical representation of the original query. Amazon Redshift then inputs this query tree into the query optimizer.
- The optimizer evaluates and if necessary rewrites the query to maximize its efficiency. This process sometimes results in creating multiple related queries to replace a single one.
- The optimizer generates a query plan (or several, if the previous step resulted in multiple queries) for the execution with the best performance. The query plan specifies execution options such as join types, join order, aggregation options, and data distribution requirements.
- You can use the EXPLAIN command to view the query plan. The query plan is a fundamental tool for analyzing and tuning complex queries.
The execution engine translates the query plan into steps, segments and streams:
Step
-
- Each step is an individual operation needed during query execution. Steps can be combined to allow compute nodes to perform a query, join, or other database operation.
Segment
-
- A combination of several steps that can be done by a single process, also the smallest compilation unit executable by a compute node slice. A slice is the unit of parallel processing in Amazon Redshift. The segments in a stream run in parallel.
Stream
-
- A collection of segments to be parcelled out over the available compute node slices.
- The execution engine generates compiled C++ code based on steps, segments, and streams. Compiled code executes faster than interpreted code and uses less compute capacity. This compiled code is then broadcast to the compute nodes.
- The compute node slices execute the query segments in parallel. As part of this process, Amazon Redshift takes advantage of optimized network communication, memory, and disk management to pass intermediate results from one query plan step to the next, which also helps to speed query execution.
- Steps 5 and 6 happen once for each stream. The engine creates the executable segments for one stream and sends them to the compute nodes. When the segments of that stream are complete, the engine generates the segments for the next stream. In this way, the engine can analyze what happened in the prior stream (for example, whether operations were disk-based) to influence the generation of segments in the next stream.
- When the compute nodes are done, they return the query results to the leader node for final processing. The leader node merges the data into a single result set and addresses any needed sorting or aggregation. The leader node then returns the results to the client.
Result Caching
-
- To reduce query execution time and improve system performance, Amazon Redshift caches the results of certain types of queries in memory on the leader node.
- When a user submits a query, Amazon Redshift checks the results cache for a valid, cached copy of the query results. If a match is found in the result cache, Amazon Redshift uses the cached results and doesn’t execute the query. Result caching is transparent to the user.
- Result caching is enabled by default. To disable result caching for the current session, set the enable_result_cache_for_session parameter to off.
- Amazon Redshift uses cached results for a new query when all of the following are true:
- The user submitting the query has access privilege to the objects used in the query.
- The table or views in the query haven’t been modified.
- The query doesn’t use a function that must be evaluated each time it’s run, such as GETDATE.
- The query doesn’t reference Amazon Redshift Spectrum external tables.
- Configuration parameters that might affect query results are unchanged.
- The query syntactically matches the cached query.
- To maximize cache effectiveness and efficient use of resources, Amazon Redshift doesn’t cache some large query result sets. Amazon Redshift determines whether to cache query results based on a number of factors. These factors include the number of entries in the cache and the instance type of your Amazon Redshift cluster.
- To determine whether a query used the result cache, query the SVL_QLOG system view. If a query used the result cache, the source_query column returns the query ID of the source query. If result caching wasn’t used, the source_query column value is NULL.